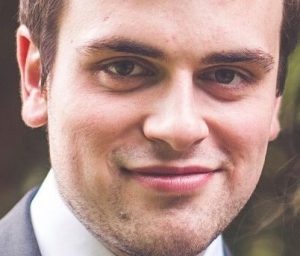
“The paradox identified by Turing and Gödel has now been brought forward into the world of AI” Matthew Colbrook (right)
“Many AI systems are unstable, and it’s becoming a major liability, especially as they are increasingly used in high-risk areas such as disease diagnosis or autonomous vehicles,” said Anders Hansen of Cambridge’s department of applied mathematics. “If AI systems are used in areas where they can do real harm if they go wrong, trust in those systems has got to be the top priority.”
Cambridge points to the work of Alan Turing and Kurt Gödel, showing that it is impossible to prove whether certain mathematical statements are true or false, that some computational problems cannot be tackled with algorithms, and some mathematical system cannot prove their own consistency. It also highlighted the 18th of 18 unsolved mathematical problems identified by Steve Smale, which concerns the limits of humans and machine intelligence.
“The paradox identified by Turing and Gödel has now been brought forward into the world of AI by Smale and others,” said fellow Cambridge researcher Matthew Colbrook (pictured). “There are fundamental limits inherent in mathematics and, similarly, AI algorithms can’t exist for certain problems.”
Developing the implications of this earlier work, the researchers say that there are cases where good neural networks can exist, yet an inherently trustworthy one cannot be built. “No matter how accurate your data is, you can never get the perfect information to build the required neural network,” said Oslo mathematician Vegard Antun. And this remains true regardless of the amount of training data available.
Not all AI is inherently flawed, and the Cambridge-Oslo team has been looking into the boundaries between reliable and unreliable AI, publishing results so far as ‘The difficulty of computing stable and accurate neural networks: On the barriers of deep learning and Smale’s 18th problem‘ in the Proceedings of the National Academy of Sciences.
“Currently, AI systems can sometimes have a touch of guesswork to them,” said Cambridge’s Hansen. “You try something, and if it doesn’t work, you add more stuff, hoping it works. At some point, you’ll get tired of not getting what you want, and you’ll try a different method. It’s important to understand the limitations of different approaches. We are at the stage where the practical successes of AI are far ahead of theory and understanding. A program on understanding the foundations of AI computing is needed to bridge this gap.”
The next step is a combine approximation theory, numerical analysis and foundations of computations to determine which neural networks can be computed by algorithms, and which can be made stable and trustworthy.
“When 20th-century mathematicians identified paradoxes, they didn’t stop studying mathematics, they just had to find new paths because they understood the limitations,” said Colbrook. “For AI, it may be a case of changing paths or developing new ones to build systems that can solve problems in a trustworthy and transparent way, while understanding their limitations.”
Tonight, I earned a spell in Facebook jail for pointing out a bug in their auto-correct software: Nobody is waiting on a “Liverpool transplant”. I’m actually beginning to regret spending a career in semiconductors, only to provide an inexpensive platform for stupid, lazy software people to act as judge and jury. Personally, I’d like to restrict Faecesbook to 1MHz, 64k DRAM and 9600 baud. This might be slow enough to allow them to catch-up, or hire an appropriate number of real humans to properly arbitrate the content of their web site. When their AI gets it wrong, do they intervene and correct/re-bias the weighting to improve the system?
Morning Neil
Not at all related to your comment…
but some self-deluding folk who make as much money as possible regardless of how many people or societies they break, shouldn’t be allowed to run companies, in my simple way of thinking.