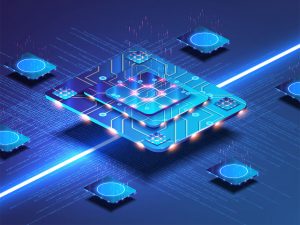
‘We have already seen that there are algorithms or neural network architectures that don’t perform as well on existing generations of accelerators, because the accelerators were designed like two years ago, and back then these neural nets didn’t exist,” says Google’s Azalia Mirhoseini, “if we reduce the design cycle, we can bridge the gap.”
After studying chip designs, the neural net can produce a design for a Google Tensor Processing Unit in less than 24 hours that beats several weeks-worth of design effort by human experts in terms of power, performance, and area.
Placement is so complex and time-consuming because it involves placing blocks of logic and memory or clusters of those blocks called macros in such a way that power and performance are maximized and the area of the chip is minimised.
Heightening the challenge is the requirement that all this happen while at the same time obeying rules about the density of interconnects. Anna Goldie and Mirhoseini targeted chip placement it takes a human expert weeks of iteration to produce an acceptable design.
Goldie and Mirhoseini modeled chip placement as a reinforcement learning problem. Reinforcement learning systems, unlike typical deep learning, do not train on a large set of labeled data.
Instead, they learn by doing, adjusting the parameters in their networks according to a reward signal when they succeed. In this case, the reward was a proxy measure of a combination of power reduction, performance improvement, and area reduction. As a result, the placement-bot becomes better at its task the more designs it does.
.