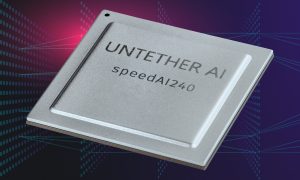
The second generation architecture also quadruples performance achieving 2Petaflops with a power budget of 30Tops per Watt.
Robert Beachler, UntetherAI’s vice president of product, told Electronics Weekly that the need for energy efficient processing is vital in AI processes because 90% of energy consumption is data movement. At-memory compute is more energy efficient that von Neumann architectures, the first generation architecture achieved 8Tops/W, which is combined with more than 1,400 RISC-V processors with custom instructions, and a new floating point (FP8) or BF16 floating point datatype for accuracy and throughput.
There are four models planned for release, the first is the speedAI240 with an array of 729 memory banks using RISC-V. It is, said Beachler, the most RISC-V on a monolithic die. The choice was a “no brainer” he explained, now that the architecture is mature and with a sufficient ecosystem available. It allows custom instructions to be implemented and delivers 2Petaflops (FP8 or 1Petaflop BF16) compute performance. For example, said UntetherAI, it can run at over 750 queries/s/W or 15 times greater than the current leading GPUs.
Each memory bank has 512 processing elements with direct attachment to dedicated SRAM. These are arranged in eight rows of 64 processing elements, with a dedicated controller for each row to allow flexibility in programming and efficient
computation of transformer network functions (e.g., Softmax and LayerNorm). The rows are managed by two RISC-V processors with over 20 custom instructions designed for inference acceleration.
The at-memory compute architecture is designed to solve problems that cannot use deterministic computing and where accuracy is critical and allow applications to run neural networks faster using AI. An obvious application is autonomous vehicles but other target applications are financial trading, sentiment analysis or speech to text translation, natural language processing as well as smart city and retail applications.
The memory architecture is also designed for scalability, with 238MB of SRAM dedicated to the processing elements for 1PB/s of memory bandwidth, four 1MB scratchpads, and two 64-bit wide ports of LPDDR5, providing up to 32GB of external DRAM.
Host and chip-to-chip connectivity is provided by high-speed PCIExpress Gen5 interfaces.
Software development support includes the imAIgine software development kit which allows push-button quantisation, optimisation, physical allocation and multi-chip partitioning. It also provides a visualisation toolkit, cycle-accurate simulator and an easily integrated runtime API.
The four speedAI devices will be offered as standalone chips as well as m.2 and PCI-Express form factor cards.
The initial offering, the speedAI240 devices and cards, will be sampling in the first half of next year, with the others to be announced and released in the second half of 2023.
Static RAM is a wonderful thing.