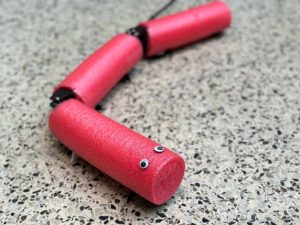
Dubbed ‘MaxDiff RL’ (maximum diffusion reinforcement learning), “the algorithm’s success lies in its ability to encourage robots to explore their environments as randomly as possible in order to gain a diverse set of experiences”, according to the university. “The algorithm works so well that robots learned new tasks and then successfully performed them within a single attempt, getting it right the first time. This starkly contrasts current AI models, which enable learning through trial and error.”
The team contrasts machine-learning for disembodied systems that use large amounts of human-curated material (ChatGPT and Google Gemini/Bard), with an embodied system like a robot that has to learn from data that it has collected without external curation.
“Traditional algorithms are not compatible with robotics in two distinct ways,” said Northwestern engineering professor Todd Murphey. “First, disembodied systems can take advantage of a world where physical laws do not apply. Second, individual failures have no consequences. For computer science applications, the only thing that matters is that it succeeds most of the time. In robotics, one failure could be catastrophic.”
Data collected by a robot is gathered sequentially rather than randomly, which can add correlations that upset conventional AI algorithms.
“We figured out a mathematical way to decorrelate the experiences of robots during data gathering, and developed an optimisation procedure that enforces this decorrelation during reinforcement learning tasks,” fellow researcher Thomas Berrueta told Electronics Weekly. “At its core, this is what MaxDiff RL is: an algorithm that encourages robots to randomise their experiences during learning in order to avoid correlations. The counter-intuitive part is, that this leads to better performance and reliability – by being more random, you get more reliable performance.”
The algorithm has been simulated in computers – and not yet used in a physical system, although NoodleBot (photo) is being developed to test it in the real world.
“Across the board, robots using MaxDiff RL learned faster than the other models. They also correctly performed tasks much more consistently and reliably than others,” according to Northwestern. “Robots using the MaxDiff RL method often succeeded at correctly performing a task in a single attempt, even when they started with no knowledge.”
Details of the algorithm are available in the paper ‘Maximum diffusion reinforcement learning‘, published in Nature Machine Intelligence – payment required without a subscription.