The average adult human brain consumes about 0.3kW hours per day. The typical modern artificial neural network ‘brain’ consumes vastly more. For example, it has been reported that training OpenAI’s GPT-3 model, with 175 billion parameters, consumes about 1.3GW hours. Using $40 per MW hour (the approximate cost of onshore wind energy), this is $52,000 in electricity operating costs alone. Next-generation large language models (LLMs) are expected to have up to one trillion parameters. Parameter count is a good proxy for training time and compute cycles and it is easy to see how generative AI (genAI) model training energy costs (and environmental impacts) are trending.
GenAI processor power consumption spirals
What is fundamentally driving this escalating power consumption? GenAI models are trained on supercomputers with thousands of accelerator modules using chiplet-based processors typically comprising a massively parallel mathematics processor and high bandwidth memories, the latest generation of which have continuous thermal design power (TDP) specifications up to 1,200W (Figure 1). The short-term peak power consumption demands of these training processors can be 2,000W or more. It is common to see three power domains used in these chiplet arrays – core, memory, and auxiliary – with the core voltage set around 0.65V for the 4nm CMOS process fabrication technology used to manufacture these 100bn transistor chips. Continuous current consumption easily tops 1,000A. The result is that genAI models require a large current and generate a sizeable amount of heat.
Technical challenges of leakage current
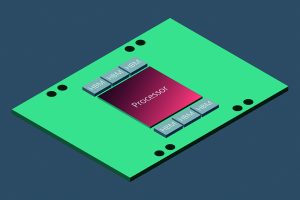
Figure 1: GenAI training GPU-based processor chiplet array with high bandwidth memories mounted on an accelerator module
CMOS transistors have two general sources of power dissipation – non-switching static (PS) and switching dynamic (PD). As the transistor size shrinks with successive generations of photolithography, its gate oxide insulator becomes thinner. Subsequently, ‘subthreshold’ and other types of leakage current, from source to drain across the gate oxide, multiplied by the transistor threshold voltage, becomes the dominant contributor to total (PS + PD) power dissipation (Figure 2).
Although measured in nA, generic CMOS transistor leakage, multiplied by many 10s of billions of transistors, dominates genAI training processor power dissipation.
Static leakage accounts for substantially more than 50% of the total processor power consumed by 4nm CMOS processors. This trend is expected to accelerate with next-generation process technology nodes, representing a huge technical challenge for the semiconductor industry. Essentially, these enormous training processors (packaging for which measures about 50x60mm) are exceptional little heaters, generally needing liquid cooling to avoid self-destruction.
PoL converters for power reductions
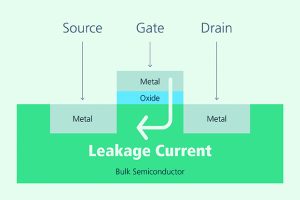
Figure 2: Simplified illustration of generic CMOS transistor leakage current
Electrical engineers have designed power supplies for microchip computer processing units for decades, typically without much consideration of or concern for dynamic performance characteristics. Until relatively recently processors have been air-cooling, using thermal conduction and convection methods. More powerful (and far less noisy) fans evolved, and TDP values for even the most advanced CPU chips were 200W or less. The dynamic current requirements (due to algorithmic loading) of CPUs and GPUs prior to 2020 were limited, but this changed around 2016, with the introduction of GPUs for AI model training and machine learning applications with TDPs of 300-450W.
The levels of TDPs and the realisation that thousands of GPUs would be needed to train emergent large language, deep-learning artificial neural network models meant that awareness around power delivery efficiency, PCB resistive power losses and undesirable supply voltage gradients across very large die areas started to increase rapidly.
Since about 2016 CMOS manufacturing process technologies have evolved from 16nm nodes to 4nm nodes, with the core logic supply voltage dropping from around 0.8VDD to around 0.65VDD, placing tighter demands on precise regulation. Given that these genAI training processor accelerator modules can cost about $30,000 each, no one wants to think about accelerator module failures caused by poor power delivery components.
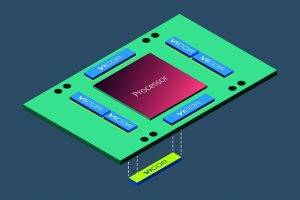
Figure 3: GenAI acceleration module with both lateral (top side) and vertical (back side) power delivery reduces PDN losses
From around 2017, in the first generations of high-current genAI processor power architectures, the point-of-load (PoL) converters were physically placed lateral (adjacent) to the processor package. The power delivery network (PDN) of a laterally-placed PoL has a lumped electrical impedance that is perhaps 200μΩ or higher, due to the resistivity of copper and the length of the traces within the PCB. As continuous current requirements for genAI training processor increase to 1,000A, this results in 200W of power dissipated in the PCB itself. When multiplied by the thousands of modules used in genAI supercomputers for LLM training, this loss becomes substantial, particularly considering that the accelerator modules are almost never powered down. GenAI accelerator modules in genAI data centres are typically running hot 24/7 for 10 years or more.
In recognition of this wasted energy, AI computer designers have begun to evaluate the placement of the PoL converters directly below the processor package, in a vertical power delivery (VPD) structure. In a VPD PDN, the lumped impedance might be 10μΩ or less, which dissipates 10W at 1,000A continuous for the core voltage domain. Moving from lateral to vertical converter placement therefore saves 190W in PCB power dissipation (200W minus 10W).
Using public domain demand forecasts of accelerator modules in the coming years (that is, more than 2.5 million units in 2024), and using reasonable estimates of the economic cost of electrical power ($40 per MWh), this 190W savings per module leads to TWh savings globally by 2027. This is equivalent to billions of dollars of electrical operating costs and millions of tons of carbon dioxide emissions reductions (depending on the renewable energy mix). These savings are annually and in perpetuity.
This era of generative AI for the IT revolution means the electronics industry has seen rapid evolution in genAI model training processor power delivery architectures. Factorised PoL converters can help to improve genAI processor power efficiency to better align genAI power consumption with societal-level environmental and conservation goals.