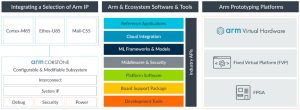
Named Ethos-U85 and configurable with between 128 and 2,048 MACs, it is intended to have enough performance to locally run heavy AI algorithms such as ‘transformers’, as well as convolutional neural networks.
“Transformer networks will drive new applications, particularly in vision and generative AI use
cases for tasks like understanding videos, filling in missing parts of images or analysing data from
multiple cameras for image classification and object detection,” according to Arm.
Its tool chain provides support for AI frameworks including TensorFlow Lite and PyTorch.
The 4Top/s figure is at 1GHz with int8 data, but it will also handle int16. This compares with 1Top/s from the earlier Ethos-U65, and up to 10Top/s from Ethos-N78.
As such it is likely to be use alongside higher-end Cortex-M CPUs and lower-end Armv9 Cortex-A CPUs.
At the same time as the new neural processor, the company announced a reference design that pairs it with the highest performance Cortex-M core, the M85.
The design, Corstone-320 (see diagram), also has a Mali-C55 image processor and a new DMA controller: DMA-320.
It is intended to “deliver the performance required for voice, audio and vision, such as real-time image classification and object recognition, or enabling voice assistants with natural language translation on smart speakers”, said Arm.
Software, machine learning models and tools are included, as well as virtual hardware for code development before hardware is available.
Ethos-U85 is expected to be in ICs next year, said Arm general manger of IoT Paul Williamson, and applications could span ‘small library language models’ – local language identification rather than local keyword identification, and industrial vision-based defect detection using transformer networks.